Building AI apps is even easier with AWS Bedrock, pre-trained models for developers to speed up their projects
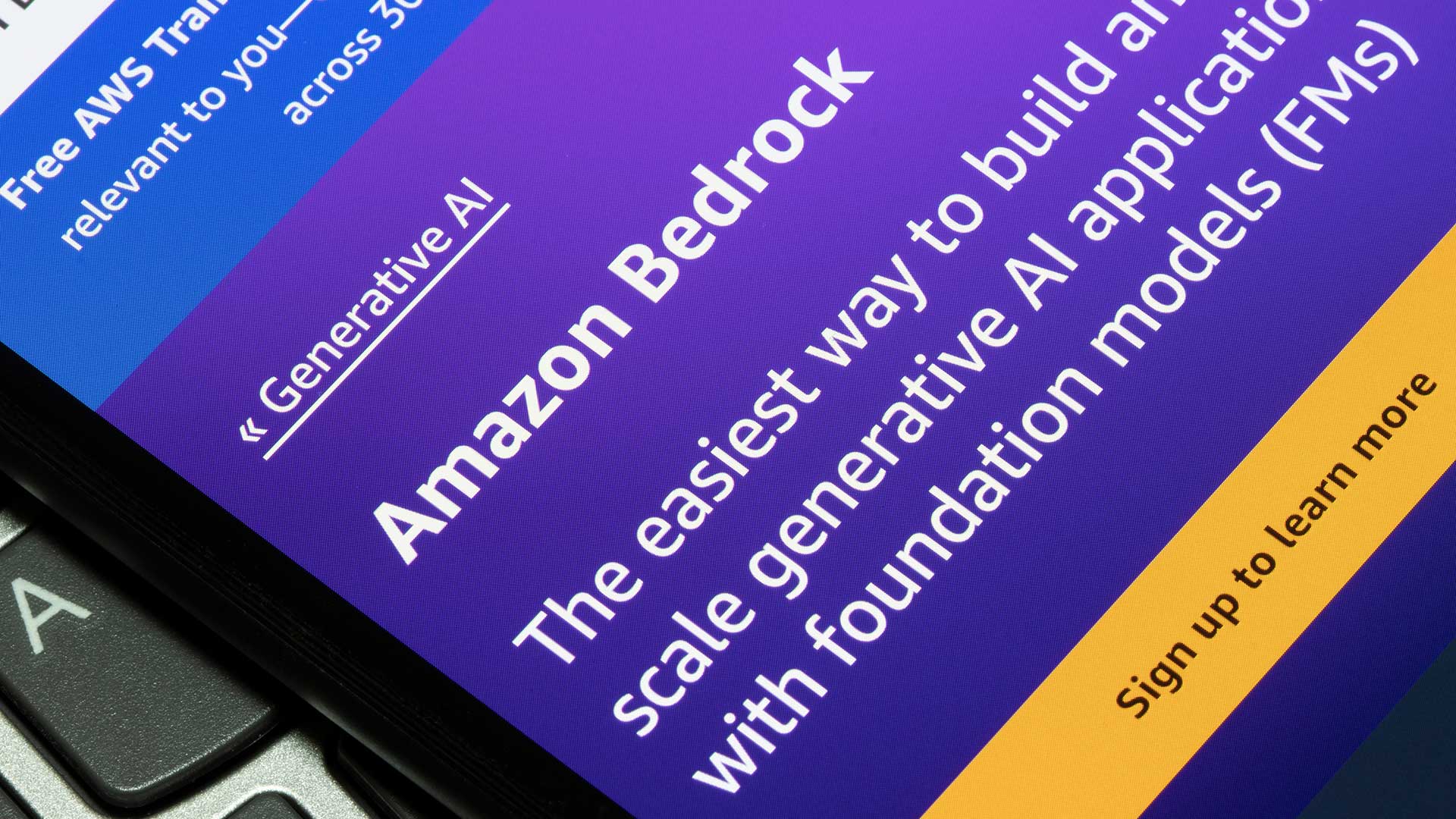
Vadim Atamanenko, Head of Analytics Reporting Department at Freedom Finance, told Global CIO about new Amazon’s service for creating AI applications: how it will simplify the work of a developer and why such tools do not make programmers unclaimed. Vadim has over 20 years of experience in software development and IT management but continues to be inspired by innovations. Let's delve into what AWS Bedrock represents.
AWS Bedrock is a new service from Amazon. This service offers pre-trained base models for building generative AI applications. The service will be useful for programmers who plan to effectively assemble applications using AI.
Bedrock provides pre-trained models optimized for specific tasks such as natural language processing (NLP), speech recognition, and computer vision. These models are built on advanced AI techniques such as deep learning and reinforcement learning and trained on large datasets. In turn, developers can significantly reduce the time to build complex applications that use AI.
The two main benefits of using Bedrock allow you to:
- Significantly reduce the time required to build and train AI models. This is especially useful for developers who work on tight deadlines or have limited resources.
- Get base models from Bedrock that are already optimized for specific tasks, developers can be sure they are starting with a solid foundation for their AI applications.
AWS Bedrock is also designed to be highly scalable, which means it can handle large amounts of data and can be used to build applications that serve millions of users. In addition, Bedrock provides APIs that allow developers to easily integrate these models into their applications. Examples of how Bedrock can be used include building chatbots and computer vision applications. For example, chatbot developers can start with a pre-trained NLP model from Bedrock and then use it to build a chatbot that can understand and respond to natural language queries. Similarly, developers building a computer vision application can start with a pre-trained computer vision model from Bedrock and then customize it to suit their specific needs.
It is important to note that while Bedrock can be a powerful tool for building AI applications, it is not a substitute for a deep understanding of AI and its underlying technologies. Developers still need to have the necessary skills and experience to build effective AI applications. Also, the base models provided by Bedrock may not be suitable for all applications, and developers may still need to develop and train their own models for certain tasks.
AWS Bedrock is a powerful tool for software developers building AI applications. By providing task-optimized pre-trained generative models, Bedrock can significantly reduce the time and effort required to build complex AI applications. Examples of how Bedrock can be used include chatbots and computer vision applications, and Bedrock's technical details include a wide range of models, advanced AI techniques, and scalability.
In recent years, the term “Internet of Things” (IoT) has become one of the most popular in the tech world. It promised to connect billions of devices. The next stage was the Industrial Internet of Things (IIoT), which brought revolutionary changes to production processes. Today, a new term is coming onto the scene – AIoT (Artificial Intelligence of Things), combining the capabilities of the Internet of Things with the power of AI.
Sixty-eight IT executives from 15 countries, including Brazil, India, USA, Saudi Arabia, Turkey, Kazakhstan, and Indonesia participated in the project.
June 2024 was a very dynamic month for AI and data analytics market, marked by significant events, product launches, and industry insights.
Artem Kalivanov, CIO of Beeline Uzbekistan, discussed IT project management in terms of project definition, management models like Waterfall and Agile methodologies, and the importance of Lean in process management.